38 federated learning with only positive labels
Federated Learning with Positive and Unlabeled Data | DeepAI Therefore, existing PU learning methods can be hardly applied in this situation. To address this problem, we propose a novel framework, namely Federated learning with Positive and Unlabeled data (FedPU), to minimize the expected risk of multiple negative classes by leveraging the labeled data in other clients. Challenges and future directions of secure federated ... Yu F X, Rawat A S, Menon A K, Kumar S. Federated learning with only positive labels. 2020, arXiv preprint arXiv: 2004.10342. Kairouz P, McMahan H B, Avent B, Bellet A, Bennis M, Bhagoji A N, Bonawitz K, Charles Z, Cormode G, Cummings R, et al. Advances and open problems in federated learning. 2019, arXiv preprint arXiv: 1912.04977
Federated Learning with Only Positive Labels To address this problem, we propose a generic framework for training with only positive labels, namely Federated Averaging with Spreadout (FedAwS), where the server imposes a geometric regularizer after each round to encourage classes to be spreadout in the embedding space.
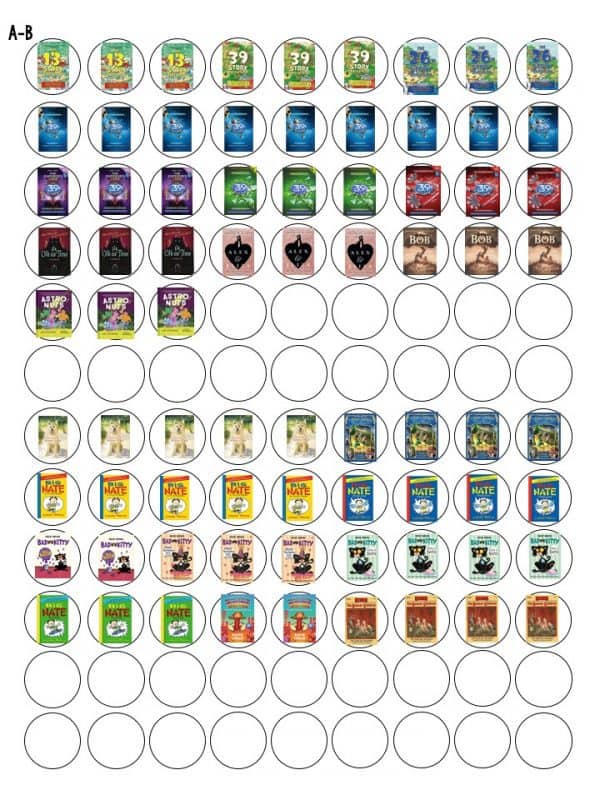
Federated learning with only positive labels
Federated Learning with Only Positive Labels | Request PDF To address this problem, we propose a generic framework for training with only positive labels, namely Federated Averaging with Spreadout (FedAwS), where the server imposes a geometric regularizer... Federated Learning with Extreme Label Skew: A Data ... Download Citation | On Jul 18, 2021, Saheed A. Tijani and others published Federated Learning with Extreme Label Skew: A Data Extension Approach | Find, read and cite all the research you need on ... Federated Learning with Only Positive Labels Federated Learning with Only Positive Labels Poster Federated Learning with Only Positive Labels Felix Xinnan Yu · Ankit Singh Rawat · Aditya Menon · Sanjiv Kumar Keywords: [ Learning Theory ] [ Parallel and Distributed Learning ] [ Privacy-preserving Statistics and Machine Learning ] [ Supervised Learning ] [ Abstract ]
Federated learning with only positive labels. A survey on federated learning - ScienceDirect This section summarizes the categorizations of federatedlearning in five aspects: data partition, privacy mechanisms, applicable machine learning models, communication architecture, and methods for solving heterogeneity. For easy understanding, we list the advantages and applications of these categorizations in Table 1. Table 1. Federated Learning with Only Positive Labels | Papers With ... To address this problem, we propose a generic framework for training with only positive labels, namely Federated Averaging with Spreadout (FedAwS), where the server imposes a geometric regularizer after each round to encourage classes to be spreadout in the embedding space. Felix X. Yu, Google Research Federated learning with only positive labels, ICML 2020 Pre-training tasks for embedding-based large-scale retrieval, ICLR 2020 Learning discrete distributions: user-lever vs item-level privacy, NeurIPS 2020 Semantic label smoothing for sequence to sequence problems, EMNLP 2020 ICML2020 Federated Learning 解读 - 3/5 - 知乎 - Zhihu From Local SGD to Local Fixed Point Methods for Federated Learning; Federated Learning简介请前往: 本系列的上一篇文章请前往: 今天我们来看这一篇: Federated Learning with Only Positive Labels. 这篇文章想要实现什么目标? 这篇文章的题目很有意思,什么是"only positive labels"?
Federated Learning with Only Positive Labels To address this problem, we propose a generic framework for training with only positive labels, namely Federated Averaging with Spreadout (FedAwS), where the server imposes a geometric regularizer after each round to encourage classes spread out in the embedding space. Federated Learning with Only Positive Labels | DeepAI To address this problem, we propose a generic framework for training with only positive labels, namely Federated Averaging with Spreadout (FedAwS), where the server imposes a geometric regularizer after each round to encourage classes to be spreadout in the embedding space. Machine learning with only positive labels - Signal ... 4 Suppose I have a binary classification problem with 10 features and about 1000 samples. In the training set, most of my data is unlabeled (75%). The rest of the data is labeled but contains only positive labels. In the test set, I have both negative and positive labels. How should I approach this classification problem? machine-learning Share GitHub - Wingspeg/FederatedLearning Federated Learning with Only Positive Labels: Google Research: Video: From Local SGD to Local Fixed-Point Methods for Federated Learning: Moscow Institute of Physics and Technology; KAUST: Slide Video: Acceleration for Compressed Gradient Descent in Distributed and Federated Optimization: KAUST: Slide Video: ICML 2019
Federated Learning with Positive and Unlabeled Data - NASA/ADS Therefore, existing PU learning methods can be hardly applied in this situation. To address this problem, we propose a novel framework, namely Federated learning with Positive and Unlabeled data (FedPU), to minimize the expected risk of multiple negative classes by leveraging the labeled data in other clients. Reading notes: Federated Learning with Only Positive Labels Authors consider a novel problem, federated learning with only positive labels, and proposed a method FedAwS algorithm that can learn a high-quality classification model without negative instance on clients Pros: The problem formulation is new. The author justified the proposed method both theoretically and empirically. Federated Contrastive Learning for Decentralized Unlabeled ... Abstract. A label-efficient paradigm in computer vision is based on self-supervised contrastive pre-training on unlabeled data followed by fine-tuning with a small number of labels. Making practical use of a federated computing environment in the clinical domain and learning on medical images poses specific challenges. GitHub - albarqouni/Federated-Learning-In-Healthcare: A ... A list of top federated deep learning papers published since 2016. Papers are collected from peer-reviewed journals and high reputed conferences. However, it might have recent papers on arXiv. A meta-data is required along the paper, e.g. topic. Some fundamental papers could be listed here as well. List of Journals / Conferences (J/C):
Identification of Tumor-Specific MRI Biomarkers Using Machine ... Apr 21, 2021 · 2. Imaging Biomarkers. Biomarker stands for biological marker and it is defined by the U.S. Food and Drug Administration (FDA) as “a defined characteristic that is measured as an indicator of normal biological processes, pathogenic processes, or responses to an exposure or intervention, including therapeutic interventions” [].
Federated Learning with Only Positive Labels. | OpenReview To address this problem, we propose a generic framework for training with only positive labels, namely Federated Averaging with Spreadout (FedAwS), where the server imposes a geometric regularizer after each round to encourage classes to be spreadout in the embedding space.
GitHub - Aryia-Behroziuan/neurons: An ANN is a model based on a collection of connected units or ...
[2004.10342] Federated Learning with Only Positive Labels [Submitted on 21 Apr 2020] Federated Learning with Only Positive Labels Felix X. Yu, Ankit Singh Rawat, Aditya Krishna Menon, Sanjiv Kumar We consider learning a multi-class classification model in the federated setting, where each user has access to the positive data associated with only a single class.
Federated learning with only positive labels - Google Research To address this problem, we propose a generic framework for training with only positive labels, namely Federated Averaging with Spreadout (FedAwS), where the server imposes a geometric regularizer after each round to encourage classes to be spreadout in the embedding space.
Positive and Unlabeled Federated Learning | OpenReview Therefore, existing PU learning methods can be hardly applied in this situation. To address this problem, we propose a novel framework, namely Federated learning with Positive and Unlabeled data (FedPU), to minimize the expected risk of multiple negative classes by leveraging the labeled data in other clients.
PDF Federated Learning with Only Positive Labels Federated Learning with Only Positive Labels However, conventional federated learning algorithms are not directly applicable to the problem of learning with only pos- itive labels due to two key reasons: First, the server cannot communicate the full model to each user. Besides sending the instance embedding model g
Federated Learning with Only Positive Labels - CORE Reader Federated Learning with Only Positive Labels - CORE Reader. We are not allowed to display external PDFs yet. You will be redirected to the full text document in the repository in a few seconds, if not click here.
Logistic Regression Implementation in Python | by Harshita ... May 14, 2021 · Logistic regression comes under the supervised learning technique. It is a classification algorithm that is used to predict discrete values. Logistic regression is based on the concept of probability.
正类标签的联邦学习(Federated Learning with Only Positive Labels)_联邦 ... 正类标签的联邦学习(Federated Learning with Only Positive Labels) 联邦学习的道路上 于 2021-07-20 15:39:54 发布 250 收藏 分类专栏: 联邦学习 文章标签: ai python
COMP 6211G: Federated Learning (Spring 2021) - iSING Lab Federated Learning with Only Positive Labels: Jiaxin Bai - Su Ying: 03/02/2021: 5: FedED: Federated Learning via Ensemble Distillation for Medical Relation Extraction: Tiezheng Yu - Wenliang Dai: 03/04/2021: 5: Resource Allocation for Wireless Federated Edge Learning based on Data Importance: Xiuzhu WANG - Linping QU: 03/04/2021: 5
[2106.10904v1] Federated Learning with Positive and ... Federated Learning with Positive and Unlabeled Data Xinyang Lin, Hanting Chen, Yixing Xu, Chao Xu, Xiaolin Gui, Yiping Deng, Yunhe Wang We study the problem of learning from positive and unlabeled (PU) data in the federated setting, where each client only labels a little part of their dataset due to the limitation of resources and time.
A survey on federated learning - ScienceDirect Federated learning is a set-up in which multiple clients collaborate to solve machine learning problems, which is under the coordination of a central aggregator. This setting also allows the training data decentralized to ensure the data privacy of each device. Federated learning adheres to two major ideas: local computing and model ...
Federated Learning with Only Positive Labels Federated Learning with Only Positive Labels Poster Federated Learning with Only Positive Labels Felix Xinnan Yu · Ankit Singh Rawat · Aditya Menon · Sanjiv Kumar Keywords: [ Learning Theory ] [ Parallel and Distributed Learning ] [ Privacy-preserving Statistics and Machine Learning ] [ Supervised Learning ] [ Abstract ]
Post a Comment for "38 federated learning with only positive labels"